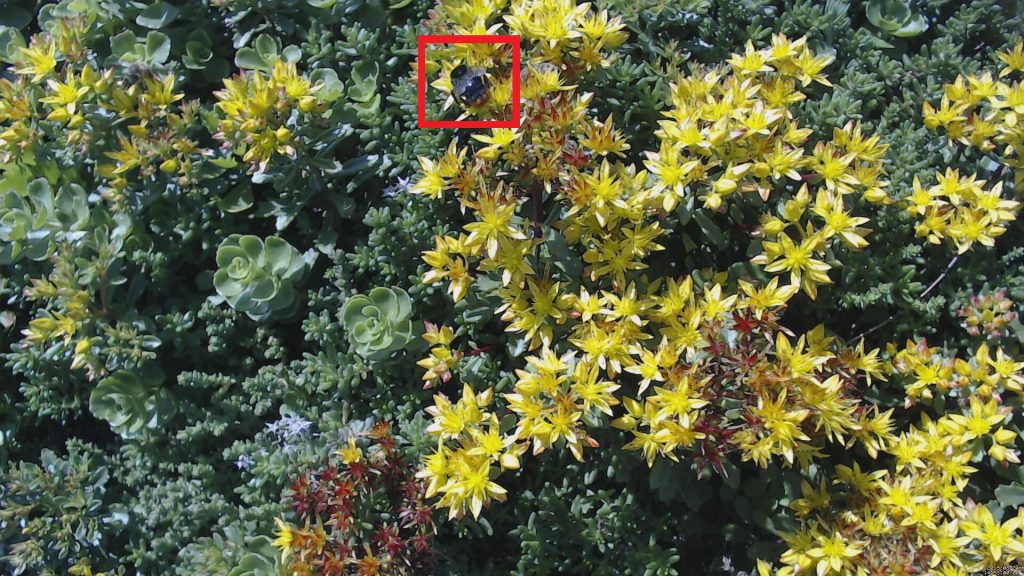
Insect benchmark datasets with time-lapse images as described in paper:
Train: train1201.zip
MD5 hash: 6831b05cab0988743a113819eb23be75
Zipped size: 8.8 GB
Unzipped size: 41.5 GB
Files: 43.683
Val: val1201.zip
MD5 hash: 88317db11fd10fab4976edb4d8d4a71f
Zipped size: 1.1 GB
Unzipped size: 5.24 GB
Files: 5.708
Test: test1201.zip
MD5 hash: d940cac65cf067a3baf356ecaa9944e3
Zipped size: 2.83 GB
Unzipped size: 12.9 GB
Files: 14.590
Labels in YOLO format: ultralytics/yolov5: label format
The annotated training and validation datasets contains insects of nine different species as listed below:
0 Coccinellidae septempunctata |
1 Apis mellifera |
2 Bombus lapidarius |
3 Bombus terrestris |
4 Eupeodes corolla |
5 Episyrphus balteatus |
6 Aglais urticae |
7 Vespula vulgaris |
8 Eristalis tenax |
The test dataset contains additional classes of insects.
9 Non-Bombus Anthophila |
10 Bombus spp. |
11 Syrphidae |
12 Fly spp. |
13 Unclear insect |
14 Mixed animals: —————————— Rhopalocera Non-Anthophila Hymenoptera Non-Syrphidae Diptera Non-Conccinalidae Coleoptera Concinellidae Other animals |
There are two naming conventions for image (.jpg) and label (.txt) files.
Background images without insects are named:
“X_Seq-YYYYMMDDHHMMSS-snapshot”.
E.g.:
Background image: 12_13-20190704172200-snapshot.jpg
Empty label file: 12_13-20190704172200-snapshot.txt
Images annotated with insects are named:
“SZ_IP-MonthDate_C_Seq-YYYYMMDDHHMMSS”.
E.g.:
Image file: S1_146-Aug23_1_156-20190822133230.jpg
Label file: S1_146-Aug23_1_156-20190822133230.txt
Abbreviations:
YYYYMMDDHHMMSS – Capture timestamp with year, month, date, hour, minutes, and second
Seq – Sequence number created by the motion program to separate images
C – Identification of two cameras with Id=0 or Id=1 in system identified by SZ_IP
MonthDate – Folder name for where the original image were stored in the system
SZ_IP – Identification of five camera systems: S1_123, S2_146, S3_194, S4_199, S5_187 (Two cameras in each system)
X – An index number related to a specific camera and folder ensuring unique file names of background images from different camera systems.
The important information in a filename is system (SZ_IP), camera Id (C) and timestamp (YYYYMMDDHHMMSS).
The three best YOLOv5 models from the paper are available in pytorch format.
All models are tested with YOLOv5 release v7.0 (22-11-2022): ultralytics/yolov5: YOLOv5 🚀 in PyTorch
Download the YOLOv5models.zip containing the files listed below.
MD5 hash: bc2194e94bfbe0ba93e4a66df6eb6f1b
Zipped size: 489 MByte
Unzipped size: 528 MByte
insect1201-bestF1-640v5m.pt: Model no. 6 in Table 2 (F1=0.912)
insect1201-bestF1-1280v5m6.pt: Model no. 8 in Table 2 (F1=0.925)
insect1201-bestF1-1280v5m6.pt: Model no. 10 in Table 2 (F1=0.932)
insects-1201val.yaml: YAML file with label names to train YOLOv5
trainInsects-1201m.sh: Linux bash shell script with parameters to train YOLOv5m6
valInsectsF1-1201.sh: Linux bash shell script with parameters to validated models