In the one year project “RGB sensor based measurements of blight in sugarbeets” (In Danish: “Sensor RGB baseret måling af bladsvampeangreb i sukkerroer “) funded by Sukkerroeafgiftsfonden (“The Sugarbeet tax fund”) and in collaboration with the Department of Agroecology, Aarhus University, a deep neural network was trained to segment images of sugarbeet. The network was trained on images collected from four strip field experiments in the 2022 growing season. Image collection was carried out using a field robot with a high quality RGB camera mounted nadir. Images were collected for 9 weeks, and a subset og images were subsequently manually annotated and used as a training set for fine-tuning the pre-trained network.
Results
The preliminary results show a good segmentation of green sugarbeet leafs (89% accuracy) and of the sugarbeet disease rust (80%), with a slight tendency to overestimate the area of the latter. The network has some trouble with distinguishing meldew from relections from the built-in flash, however, the latter was classified as green leaf in the training set rather than being given its own class.
Input image Ground truth Segmented image
Applying the network to all the collected images, show a clear pattern of the rust initially developing at a few hotspots, which continueue to develop and spread throughout the season. The infection seem to move along the stripes and to a lesser extend across them.
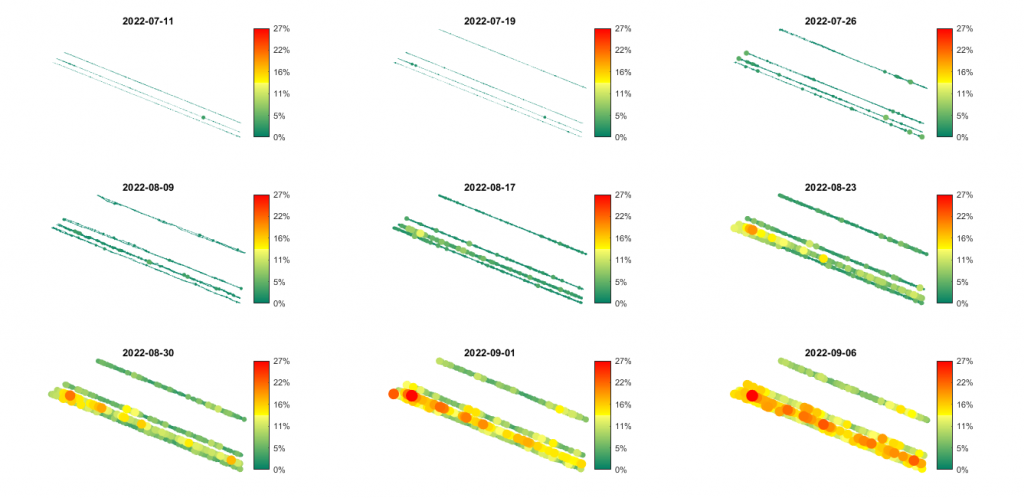
These preliminary results were published in “NBR Faglig beretning 2022” (non-peer reviewed). For more details, see “Kamera og kunstig intelligens til vurdering af sygdomstryk i sukkerroer” in “NBR Faglig beretning 2022”.
For more information, contact Anders Krogh Mortensen or René Gislum.
Data availability
The collected images are available here.