To combat weeds, farmers have been spraying their fields with herbicides for decades. Until now, however, they have used a standard herbicide mixture, which are spread over the entire field. This is not particularly smart from either a financial point of view or an environmental or biological perspective because herbicides are expensive, can have implications for the water quality in streams and groundwater, and lead to resistance in the weeds. Not to mention that a standard mixture of herbicides may have a good effect on some species of weeds, but a less effective impact on others.
Today, several Decision Support Systems exist, which can recommend optimal herbicide dosages for a given weed population in a field thereby reducing the farmer’ herbicide expenditures by at least 40%. However, in order to use this system, it is necessary to inspect the field and assess the field’s weed population.
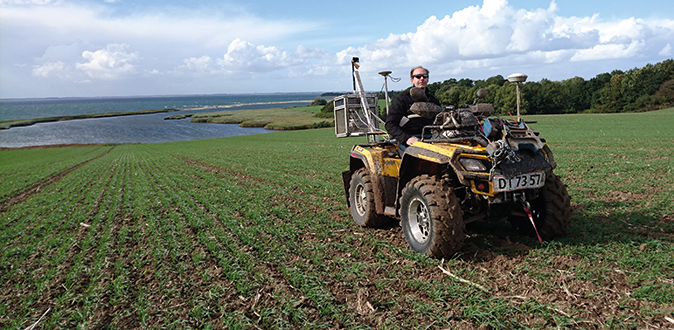
In RoboWeedMaPS the aim is to bridge the gap between the potential herbicide savings and the required weed inspections by using image analysis for automating the weed inspection part. Thus, it will only be necessary to collect images from representative parts of the field, after which the computer will determine which weeds are present in the field and which actions to take for controlling the weeds. By combining an automatic, camera-based weed recognition with a decision support tool, the farmer can get advice on the optimal use of his equipment while saving herbicide.
The images are automatically uploaded in the cloud, where they are analysed by several specialised algorithms for the composition of the weeds compared with the competitive ability of the crops. This is where Big Data come into the picture because the whole system relies on an enormous ‘weed data base’, where the researchers use deep learning to teach the computer to recognise different types of weeds.
Although it might sound simple, it is actually quite difficult to automate the process, particularly because weeds in the fields hardly ever resemble those in the weed botanical books.
The project has now reached the stage where the cameras can take high-resolution images with an accuracy of 4 pixels per millimetre, even when driving at 50 km/h in the field, and where the computer has satisfactorily learned to recognise 27 types of weeds from a data base withthousands of images. The computer is trained with far more species of weeds, but the number of training images is still too small to achieve strong recognition.
The aim is to make everyday life easier for farmers, so that the computer itself finds where the weeds are located, what type they are, and which type of herbicide should be used at precisely that spot in the field. The computer will thus control the dosage when farmers are spraying their fields, and even regulate different types of herbicides and dosages depending on the type of weed – an important part of the future smart farming.
2022
Toke T. Høye, Mads Dyrmann, Christian Kjær, Johnny Nielsen, Marianne Bruus, Cecilie L. Mielec, Maria S. Vesterdal, Kim Bjerge, Sigurd A. Madsen, Mads R. Jeppesen, Claus Melvad. (2022).
Accurate image-based identification of macroinvertebrate specimens using deep learning-How much training data is needed?. PeerJ
(Paper) 2021
Skovsen, Søren Kelstrup; Laursen, Morten Stigaard; Kristensen, Rebekka Kjeldgaard; Rasmussen, Jim; Dyrmann, Mads; Eriksen, Jørgen; Gislum, René; Nyholm Jørgensen, Rasmus; Karstoft, Henrik. (2021).
Robust Species Distribution Mapping of Crop Mixtures Using Color Images and Convolutional Neural Networks. Sensors
(Paper)
Mads Dyrmann, Anders Krogh Mortensen, Lars Linneberg, Toke Thomas Høye, Kim Bjerge. (2021).
Camera Assisted Roadside Monitoring for Invasive Alien Plant Species Using Deep Learning. Sensors
(Paper)
Mikkel Fly Kragh, Jens Rimestad, Jacob Theilgaard Lassen, Jørgen Berntsen, Henrik Karstoft. (2021).
Predicting embryo viability based on self-supervised alignment of time-lapse videos. IEEE Transactions on Medical Imaging
(Paper)
Mikkel Fly Kragh, Henrik Karstoft. (2021).
Embryo selection with artificial intelligence: how to evaluate and compare methods?. Journal of Assisted Reproduction and Genetics
(Paper)
Farkhani, Sadaf; Skovsen, Søren Kelstrup; Dyrmann, Mads; Nyholm Jørgensen, Rasmus; Karstoft, Henrik. (2021).
Weed classification using explainable multi-resolution slot attention. Sensors
(Paper) 2020
Søren K. Skovsen; Harald Haraldsson; Abe Davis; Henrik Karstoft; Serge Belongie. (2020).
Decoupled Localization and Sensing with HMD-based AR for Interactive Scene Acquisition.
(Paper) 2019
Sadaf Farkhani, Mikkel Fly Kragh, Peter Hviid Christiansen, Rasmus Nyholm Jørgensen, Henrik Karstoft. (2019).
Sparse-to-Dense Depth Completion in Precision Farming.
(Paper) 2020
Sadaf Farkhani, Søren K Skovsen, Anders K Mortensen, Morten S Laursen, Rasmus N Jørgensen, Henrik Karstoft. (2020).
Initial evaluation of enriching satellite imagery using sparse proximal sensing in precision farming.
(Paper) (Presentation, video)
Søren Kelstrup Skovsen, Morten Stigaard Laursen , Rebekka Kjeldgaard Kristensen, Jim Rasmussen, Mads Dyrmann , Jørgen Eriksen, René Gislum, Rasmus Nyholm Jørgensen, Henrik Karstoft 1. (2020).
Robust Species Distribution Mapping of Crop Mixtures Using Color Images and Convolutional Neural Networks. Sensors
(Paper) (Presentation, slides) 2019
Søren Skovsen, Mads Dyrmann, Anders K. Mortensen, Morten S. Laursen, René Gislum, Jørgen Eriksen, Sadaf Farkhani, Henrik Karstoft, Rasmus N. Jørgensen. (2019).
The GrassClover Image Dataset for Semantic and Hierarchical Species Understanding in Agriculture. The IEEE Conference on Computer Vision and Pattern Recognition (CVPR) Workshops
(Paper)
Simon Leminen Madsen, Anders Krogh Mortensen, Rasmus Nyholm Jørgensen, Henrik Karstoft. (2019).
Disentangling Information in Artificial Images of Plant Seedlings Using Semi-Supervised GAN. Remote Sensing, Volume 11.
(Paper)
Simon Leminen Madsen, Mads Dyrmann, Rasmus Nyholm Jørgensen, Henrik Karstoft. (2019).
Generating Artificial Images of Plant Seedlings using Generative Adversarial Networks. Biosystems Engineering, Volume 187.
(Paper) 2014
Mads Dyrmann, Peter Christiansen. (2014).
Automated Classification of Seedlings Using Computer Vision: Pattern Recognition of Seedlings Combining Features of Plants and Leaves for Improved Discrimination. Aarhus University
(Paper) 2019
Mikkel Fly Kragh, Jens Rimestad, Jørgen Berntsen, Henrik Karstoft. (2019).
Automatic grading of human blastocysts from time-lapse imaging. Computers in Biology and Medicine
(Paper) 2018
Nima Teimouri, Mads Dyrmann, Per Rydahl Nielsen, Solvejg Kopp Mathiassen, Gayle J. Somerville, and Rasmus Nyholm Jørgensen. (2018).
Weed Growth Stage Estimator Using Deep Convolutional Neural Networks. Sensors
(Paper)
Søren Skovsen, Mads Dyrmann, Jørgen Eriksen, René Gislum, Henrik Karstoft, Rasmus Nyholm Jørgensen. (2018).
Predicting Dry Matter Composition of Grass Clover Leys Using Data Simulation and Camera-Based Segmentation of Field Canopies into White Clover, Red Clover, Grass and Weeds. 14th International Conference on Precision Agriculture
(Paper) 2017
Søren Skovsen, Mads Dyrmann, Anders Krogh Mortensen, Kim Arild Steen, Ole Green, Jørgen Erikse, René Gislum, Rasmus Nyholm Jørgensen, and Henrik Karstoft. (2017).
Estimation of the Botanical Composition of Clover-Grass Leys from RGB Images Using Data Simulation and Fully Convolutional Neural Networks. Sensors, special issue: Sensors in Agriculture
(Paper) 2019
Nima Teimouri, Mads Dyrmann, Rasmus Nyholm Jørgensen. (2019).
A Novel Spatio-Temporal FCN-LSTM Network for Recognizing Various Crop Types Using Multi-Temporal Radar Images. Remote Sensing
(Paper)
Mikkel Kragh, James Underwood. (2019).
Multi-Modal Obstacle Detection in Unstructured Environments with Conditional Random Fields. Journal of Field Robotics
(Paper) 2018
Anders Krogh Mortensen, Asher Bender, Brett Whelan, Margaret M. Barbour, Salah Sukkarieh, Henrik Karstoft, René Gislum. (2018).
Segmentation of lettuce in coloured 3D point clouds for fresh weight estimation. Computers and Electronics in Agriculture
(Paper) 2015
Peter Christiansen, Mikkel Fly Kragh, Kim Arild Steen, Henrik Karstoft, Rasmus Nyholm Jørgensen. (2015).
Advanced sensor platform for human detection and protection in autonomous farming. 10th European Conference on Precision Agriculture (ECPA)
(Paper) 2018
Dennis Larsen, Søren Skovsen, Kim Arild Steen, Kevin Grooters, Jørgen Eriksen, Ole Green, Rasmus Nyholm Jørgensen. (2018).
Autonomous Mapping of Grass-Clover Ratio Based on Unmanned Aerial Vehicles and Convolutional Neural Networks. 14th International Conference on Precision Agriculture
(Paper) 2017
Timo Korthals, Mikkel Fly Kragh, Peter Christiansen, Ulrich Rückert. (2017).
Towards Inverse Sensor Mapping in Agriculture. International Conference on Intelligent Robots and Systems (IROS 2017) Workshop
(Paper) 2018
Martin Peter Christiansen, Morten Stigaard Laursen, Rasmus Nyholm Jørgensen, Søren Skovsen, René Gislum. (2018).
Ground Vehicle Mapping of Fields Using LiDAR to Enable Prediction of Crop Biomass. 14th International Conference on Precision Agriculture
(Paper)
Mads Dyrmann, Søren Skovsen, Morten Stigaard Laursen, and Rasmus Nyholm Jørgensen. (2018).
Using a fully convolutional neural network for detecting locations of weeds in images from cereal fields. 14th International Conference on Precision Agriculture
(Paper)
Hadi Karimi, Søren Skovsen, Mads Dyrmann, and Rasmus Nyholm Jørgensen. (2018).
A Novel Locating System for Cereal Plant Stem Emerging Points’ Detection Using a Convolutional Neural Network. Sensors
(Paper)
Nima Teimouri, M. Omid, K. Mollazade, H. Mousazadeh, R. Alimardani, H. Karstof. (2018).
On-line separation and sorting of chicken portions using a robust vision-based intelligent modelling approach. Biosystems Engineering
(Paper) 2015
Mikkel Fly Kragh, Rasmus Nyholm Jørgensen, Henrik Pedersen. (2015).
Object Detection and Terrain Classification in Agricultural Fields using 3D Lidar Data. 10th International Conference on Computer Vision Systems
(Paper) 2016
Mikkel Fly Kragh, Peter Christiansen, Timo Korthals, Thorsten Jungeblut, Henrik Karstoft, Rasmus Nyholm Jørgensen. (2016).
Multi-modal Obstacle Detection and Evaluation of Occupancy Grid Mapping in Agriculture. International Conference on Agricultural Engineering 2016
(Paper) 2017
Peter Christiansen, Mikkel Kragh, Kim A. Steen, Henrik Karstoft, Rasmus N. Jørgensen. (2017).
Platform for evaluating sensors and human detection in autonomous mowing operations. Precision Agriculture
(Paper)
Mikkel Fly Kragh, Peter Christiansen, Morten Stigaard Laursen, Morten Larsen, Kim Arild Steen, Ole Green, Henrik Karstoft, Rasmus Nyholm Jørgensen. (2017).
FieldSAFE: Dataset for Obstacle Detection in Agriculture. Sensors
(Paper)
Anders K. Mortensen, Henrik Karstoft, Karen Søegaard, René Gislum, and Rasmus N. Jørgensen. (2017).
Preliminary Results of Clover and Grass Coverage and Total Dry Matter Estimation in Clover-Grass Crops Using Image Analysis. Journal of Imaging, special issue: Remote and Proximal Sensing Applications in Agriculture
(Paper) 2012
M.R. Andersen, T. Jensen, P. Lisouski, A.K. Mortensen, M.K. Hansen, T. Gregersen, P. Ahrendt. (2012).
Kinect Depth Sensor Evaluation for Computer Vision Applications. Technical Report, Electronics and Computer Engineering, Department of Engineering, Aarhus University
(Paper) 2016
A. K. Mortensen, P. Lisouski and P. Ahrendt. (2016).
Weight prediction of broiler chickens using 3D computer vision. Computers and Electronics in Agriculture
(Paper) 2017
Rasmus Nyholm Jørgensen, Mads Dyrmann. (2017).
Automatisk ukrudtsgenkendelse er ikke længere science fiction. Plantekongres '17
(Paper)
Mads Dyrmann, Rasmus Nyholm Jørgensen, Henrik Skov Midtiby. (2017).
Detection of Weed Locations in Leaf-occluded Cereal Crops using a Fully-Convolutional Neural Network. Advances in Animal Biosciences Volume 8, Issue 2 (Papers presented at the 11th European Conference on Precision Agriculture (ECPA 2017)
(Paper)
Thomas Mosgaard Giselsson, Rasmus Nyholm Jørgensen, Peter Kryger Jensen, Mads Dyrmann, Henrik Skov Midtiby. (2017).
A Public Image Database for Benchmark of Plant Seedling Classification Algorithms. arXiv
(Paper) 2016
M. S. Laursen, R. N. Jørgensen, H. S. Midtiby, K. Jensen, M. P. Christiansen, T. M. Giselsson, A. K. Mortensen and P. K. Jensen. (2016).
Dicotyledon Weed Quantification Algorithm for Selective Herbicide Application in Maize Crops. Sensors
(Paper) 2017
Per Rydahl, Niels-Peter Jensen, Mads Dyrmann, Poul Henning Nielsen, Rasmus Nyholm Jørgensen. (2017).
Presentation of a cloud based system bridging the gap between in-field weed inspections and decision support systems. Advances in Animal Biosciences Volume 8, Issue 2 (Papers presented at the 11th European Conference on Precision Agriculture (ECPA 2017)
(Paper) 2015
Mads Dyrmann. (2015).
Fuzzy C-means based plant segmentation with distance dependent threshold. Proceedings of the Computer Vision Problems in Plant Phenotyping (CVPPP)
(Paper) 2016
Mads Dyrmann, Henrik Karstoft, Henrik Skov Midtiby. (2016).
Plant species classification using deep convolutional neural network. Biosystems Engineering
(Paper)
Mads Dyrmann, Anders Krogh Mortensen, Henrik Skov Midtiby, Rasmus Nyholm Jørgensen. (2016).
Pixel-wise classification of weeds and crops in images by using a Fully Convolutional neural network. 4th International Conference on Agricultural and Biosystems Engineering
(Paper) (Presentation, video)
Mads Dyrmann, Henrik Skov Midtiby, Rasmus Nyholm Jørgensen. (2016).
Evaluation of intra variability between annotators of weed species in color images. 4th International Conference on Agricultural and Biosystems Engineering
(Paper) 2017
Morten Stigaard Laursen, Rasmus Nyholm Jørgensen, Mads Dyrmann, Robert Poulsen. (2017).
RoboWeedSupport – Sub Millimeter Weed Image Acquisition in Cereal Crops with Speeds up till 50 Km/h. International Journal of Biological, Biomolecular, Agricultural, Food and Biotechnological Engineering (ICSWTS 2017)
(Paper)
Mads Dyrmann, Peter Christiansen. (2017).
Estimation of plant species by classifying plants and leaves in combination. Journal of Field Robotics
(Paper)
Mads Dyrmann. (2017).
Automatic Detection and Classification of Weed Seedlings under Natural Light Conditions. University of Southern Denmark
(Paper) 2016
Peter Christiansen, Lars N Nielsen, Kim A Steen, Rasmus N Jørgensen, Henrik Karstoft. (2016).
DeepAnomaly: Combining Background Subtraction and Deep Learning for Detecting Obstacles and Anomalies in an Agricultural Field. Sensors
(Paper)
Mikkel Kragh, Kim Bjerge, Peter Ahrendt. (2016).
3D impurity inspection of cylindrical transparent containers. Measurement Science and Technology
(Paper)