Safer Autonomous Farming Equipment
|
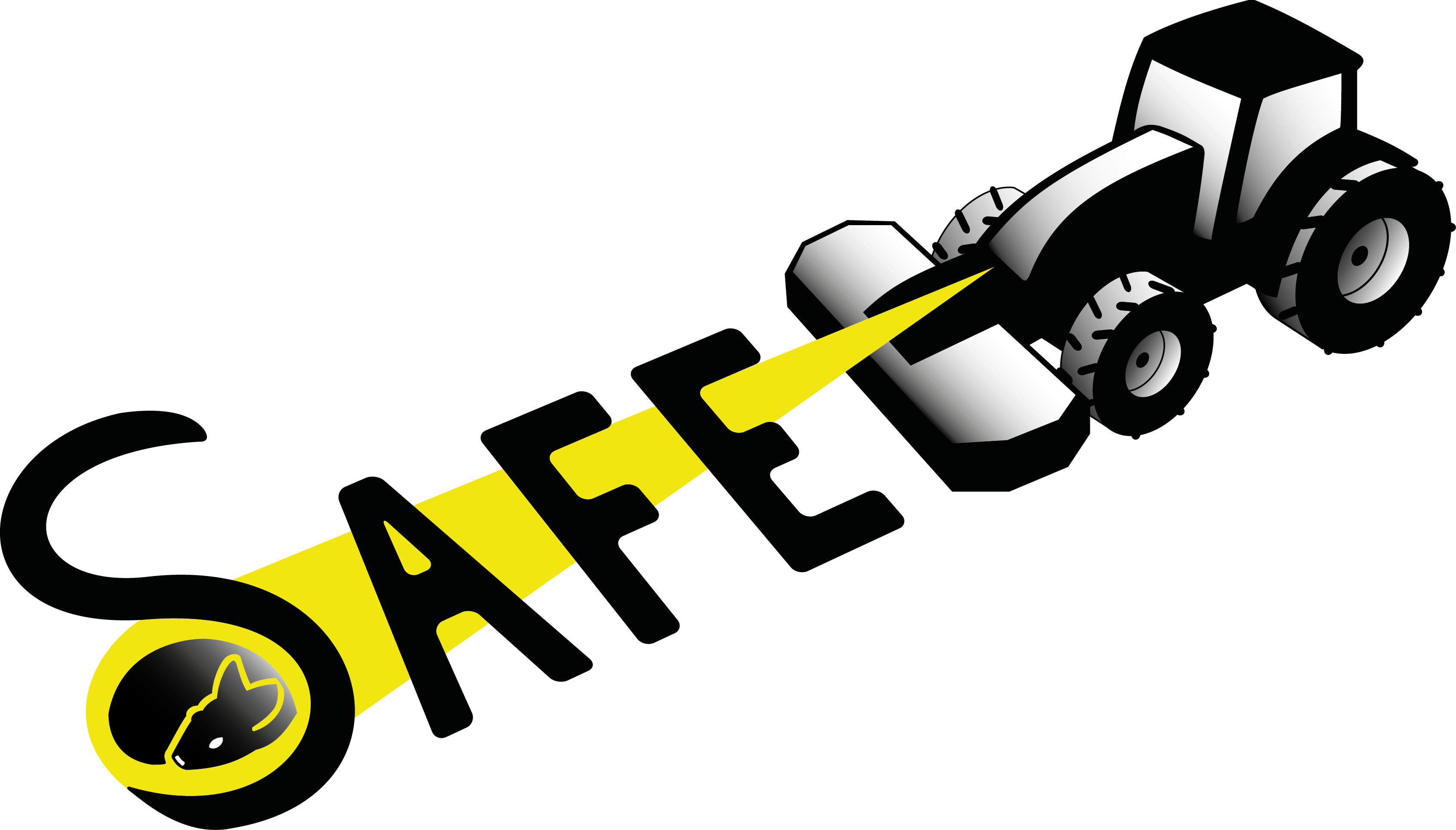 |
Automation of the agricultural sector is at the top of the agenda – both in terms of the new EU Framework program, Horizon2020, as well as the global need for more efficient usage of our food resources. Here, robotics has proven to be effective in many areas – amongst others when it comes to the increasing demands for safety within the agricultural sector which is in the process of becoming increasingly automated.
The SAFE project focuses on the safety-related issues.
Background
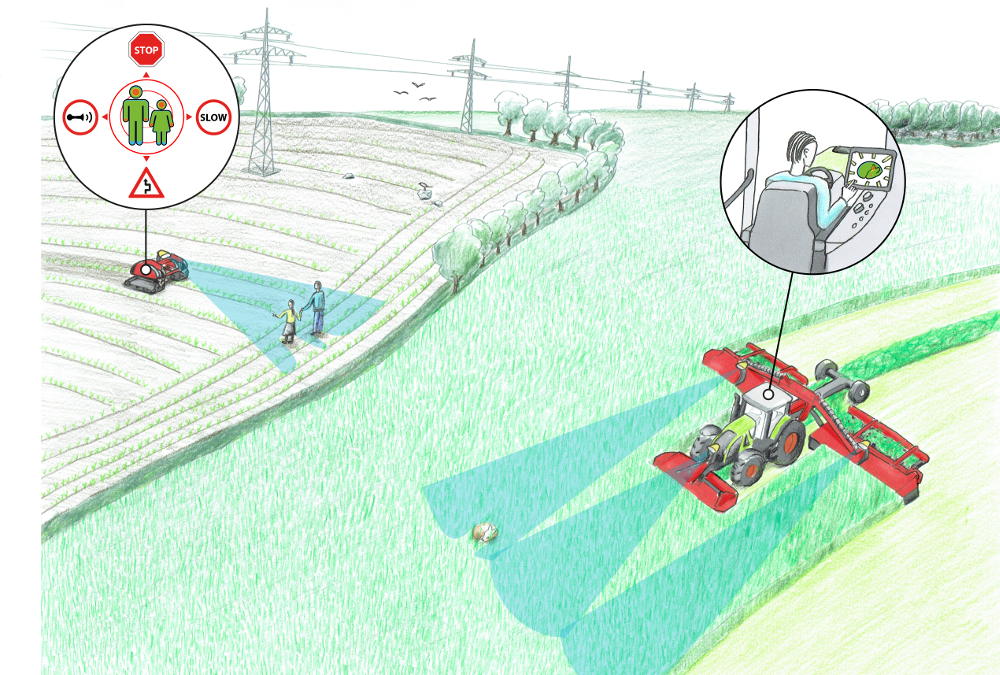
The aim of the SAFE project is to develop autonomous agricultural machinery that will be able to harvest green biomass and cultivate row crops – without animals or humans being exposed to any type of safety risk. Today, autonomous agricultural machinery is already being used in the fields. However, this machinery requires continuously monitoring in order to ensure the safety of humans and animals.
This is why the SAFE project brings together the major manufacturers of agricultural machinery and the universities in order to develop advanced sensors, perception algorithms, and rational behaviors for semi-automated tractors and implements and finally autonomous robots. This will enable the machines to detect the presence of humans and/or animals in its vicinity which will then prevent accidents and, to a much greater extent than today, prevent injuries inflicted on animals (deer, birds, etc.) hiding in the field.
Publications
2019
Sadaf Farkhani, Mikkel Fly Kragh, Peter Hviid Christiansen, Rasmus Nyholm Jørgensen, Henrik Karstoft. (2019).
Sparse-to-Dense Depth Completion in Precision Farming.
(Paper)
Mikkel Kragh, James Underwood. (2019).
Multi-Modal Obstacle Detection in Unstructured Environments with Conditional Random Fields. Journal of Field Robotics
(Paper) 2017
Timo Korthals, Mikkel Fly Kragh, Peter Christiansen, Ulrich Rückert. (2017).
Towards Inverse Sensor Mapping in Agriculture. International Conference on Intelligent Robots and Systems (IROS 2017) Workshop
(Paper)
Peter Christiansen, Mikkel Kragh, Kim A. Steen, Henrik Karstoft, Rasmus N. Jørgensen. (2017).
Platform for evaluating sensors and human detection in autonomous mowing operations. Precision Agriculture
(Paper)
Mikkel Fly Kragh, Peter Christiansen, Morten Stigaard Laursen, Morten Larsen, Kim Arild Steen, Ole Green, Henrik Karstoft, Rasmus Nyholm Jørgensen. (2017).
FieldSAFE: Dataset for Obstacle Detection in Agriculture. Sensors
(Paper) 2016
Mikkel Fly Kragh, Peter Christiansen, Timo Korthals, Thorsten Jungeblut, Henrik Karstoft, Rasmus Nyholm Jørgensen. (2016).
Multi-modal Obstacle Detection and Evaluation of Occupancy Grid Mapping in Agriculture. International Conference on Agricultural Engineering 2016
(Paper)
Peter Christiansen, Lars N Nielsen, Kim A Steen, Rasmus N Jørgensen, Henrik Karstoft. (2016).
DeepAnomaly: Combining Background Subtraction and Deep Learning for Detecting Obstacles and Anomalies in an Agricultural Field. Sensors
(Paper) 2015
Mikkel Fly Kragh, Rasmus Nyholm Jørgensen, Henrik Pedersen. (2015).
Object Detection and Terrain Classification in Agricultural Fields using 3D Lidar Data. 10th International Conference on Computer Vision Systems
(Paper)
Peter Christiansen, Mikkel Fly Kragh, Kim Arild Steen, Henrik Karstoft, Rasmus Nyholm Jørgensen. (2015).
Advanced sensor platform for human detection and protection in autonomous farming. 10th European Conference on Precision Agriculture (ECPA)
(Paper)
Datasets
Recently, we have published the FieldSAFE dataset, which is a novel multi-modal dataset for obstacle detection in agriculture.
Partners

For more information
For more information, please visit the official SAFE webpage http://safefarming.dk